Technology changes so fast; it’s hard to know the best ways to stay healthy long-term. What was once cutting-edge, quickly becomes outdated. Imagine: nutrition plans perfectly matched to you! Thanks to technology and machine learning, this is now possible.
People are really into personalized nutrition these days. Genetics, lifestyle, and gut bacteria—all this data is used by advanced machine learning to give personalized nutrition advice. Check out the food recommendations—they’re personalized based on what we know about you from this data!
Table Of Contents:
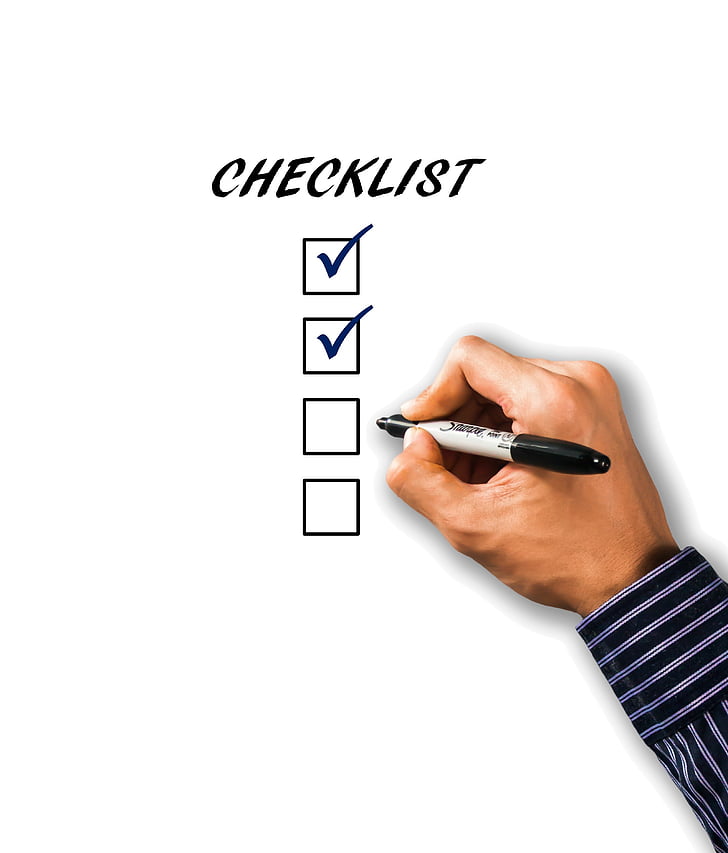
- Decoding the data: How machine learning works in nutrition
- Beyond the bite: Limitations and considerations with ML
- ML-powered nutrition advancements: practical applications
- Ethical considerations and data privacy in the age of ML-powered nutrition
- Beyond binary: Subgroup analysis and individualized treatment
- Reproducibility and replicability: This won’t be a walk in the park; there will be challenges.
- Integrating social and cultural factors: A multidisciplinary approach
- Personalizing your diet to fit your needs — how machine learning and you work together
- Hence we sum up all the advice right here!
Decoding the data: How machine learning works in nutrition
Traditional nutrition relied heavily on observational studies. Clinical trials, while valuable, can take a long time to produce actionable results.
Machine learning is great at figuring things out from information; it can spot connections between different things to predict what will happen next. Could there be a formula that connects what you eat to how you feel? These relationships? Machine learning can figure them out. I think that’s pretty rad; it’s really cool how well it works.
The standard method involves splitting the data. The first part becomes training data used to develop a predictive model.
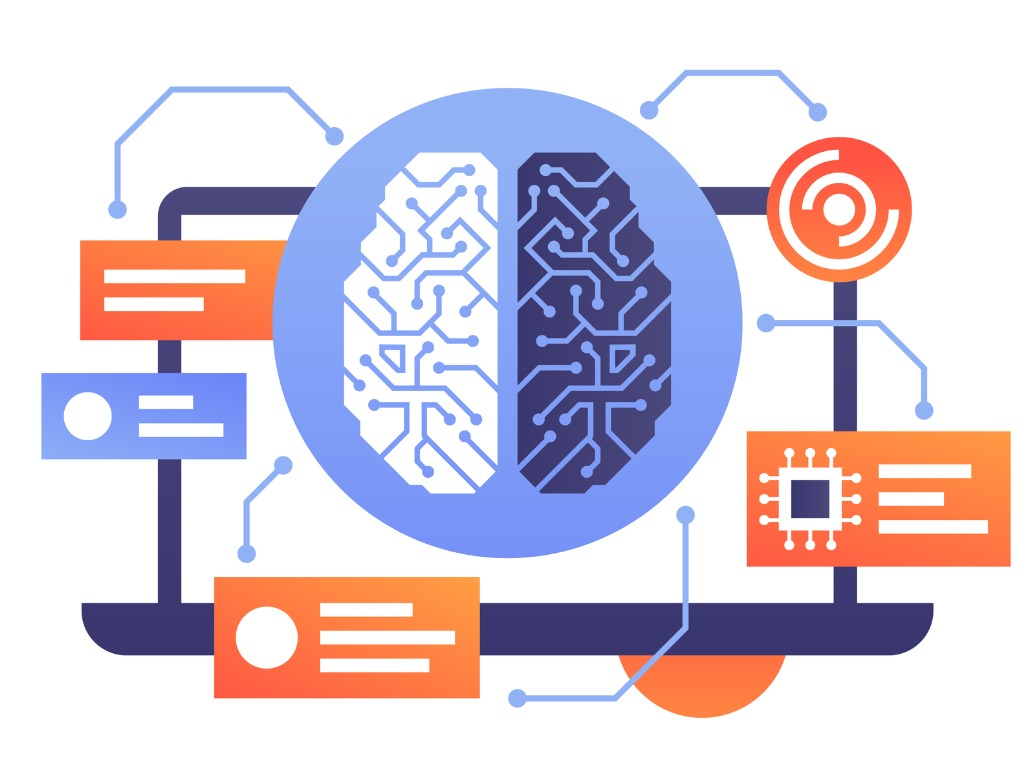
The remaining data is the “test” set. A great opportunity arose to test the prediction tool’s accuracy: a perfect dataset. It would reveal if it was a success or a failure. I’m impressed; the effectiveness was clear from the results. Those were simply great. Machine learning uses many methods; some examples include linear regression, support vector machines, decision trees, and random forests.
Machine learning in action: Real-world examples
ML techniques are already making an impact in personalized nutrition. Sixty studies on personalized diets and data appeared in scientific journals between 2014 and 2021.
Genetics isn’t the whole explanation; other influences are at play. It includes epigenomics, metabolomics, and even microbiomics (the study of microorganisms living in and on your body).
Custom solutions need a lot of information. When the data quantity becomes great, techniques that focus on selecting individual variables can become less reliable.
Digesting the possibilities: Opportunities of ML in nutrition
We’re already seeing glimpses of ML tackling these questions. People expect this field to grow in popularity and use soon.
Better computers are the reason for this growth. Institutions like the US National Institute of Health are also dedicating resources to accelerate ai development.
Beyond the bite: Limitations and considerations with ML
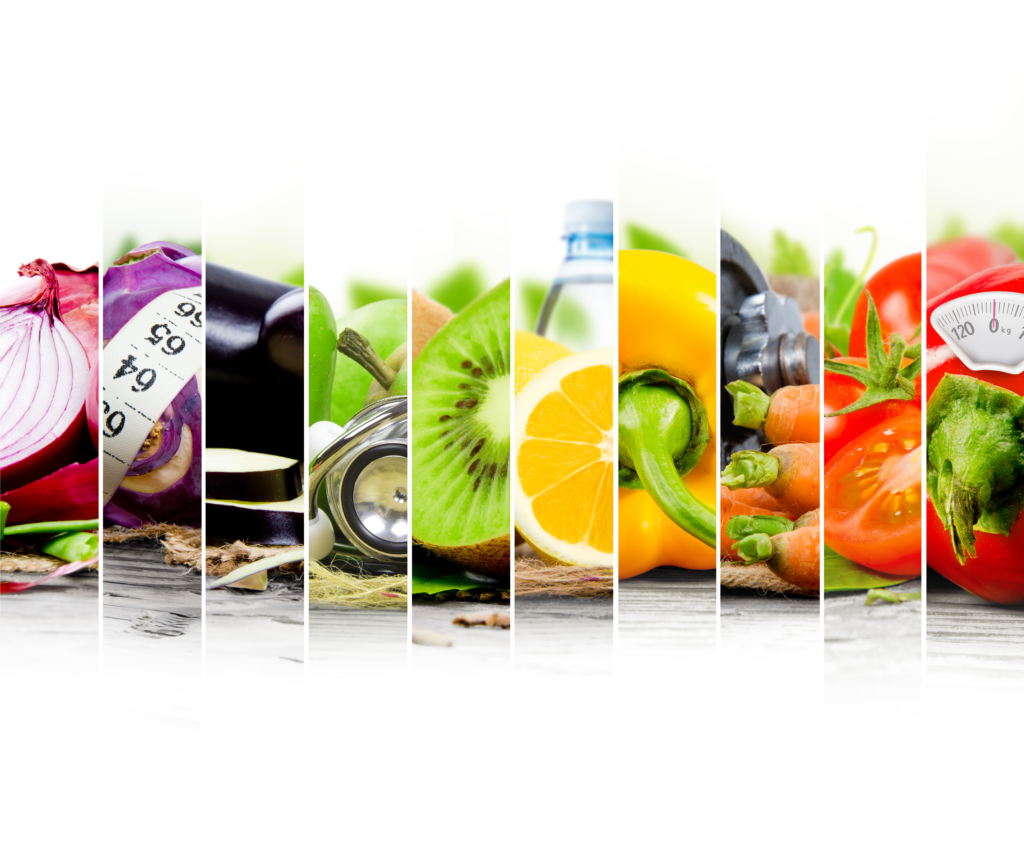
Most studies to date focus on describing data relationships. To give really good advice, we have to experiment and see which methods are most effective. Expect progress; this upgrade will improve our performance significantly. For example, are shorter, more direct recommendations better than longer, more detailed ones? What about personalized recommendations versus general ones? Addressing these questions is our next step. Progress depends on these.
The scientific method depends on the replication of results; without it, data validation is impossible. Think of it like a recipe—if you can’t make the same cake twice using the same ingredients, there’s something wrong with the recipe. If one person experiences a particular outcome and another experiences something entirely different, it is difficult to draw broad conclusions.
What counts as “replication” is different in each scientific field. How well would ML models predict outcomes?
Study participants are usually pretty familiar with healthy habits. We brought in the best—leading researchers—to plan this study.
Defining success: The problem of outcome measures
We value things like stable blood sugar levels. Using this one thing to measure health? Scientifically, we don’t have solid proof it works. The next step? More research is needed; the current data is insufficient. A lot more. Other body composition markers also lack universally defined optimal ranges.
Conflicting information, like what we see with blood sugar and soluble markers, can be a real headache. Science and nutrition pros agree. Scientific progress in all disciplines is hampered by a lack of advanced computer modeling techniques. Current tools are inadequate; better options are necessary for improved efficiency and results. Think medicine, engineering, even history – the applications are endless.
ML-powered nutrition advancements: practical applications
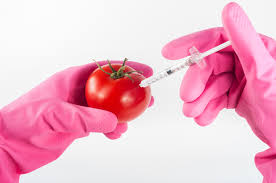
Nutrition experts and scientists participated in a coordinated discussion at the National Academies of Sciences, Engineering, and Medicine (NASEM) in Washington, D.C. The meeting’s goal? To develop workable nutrition plans—ones that people can use to make real, lasting changes to their diet and health. We talked about everything: portion sizes and picking healthier foods.
This isn’t theoretical. Real-world tools are using machine learning.
People are really embracing these new approaches to nutrition; they’re becoming quite trendy. Sales are up. Forecasts predict the industry will reach $40.07 billion by 2032.
Here are a few examples:
- BetterMeal.AI: Offers plans using a gut-focused and whole-body approach. IBD, PCOS, and thyroid issues? We’re ready to lend a hand; we’ve got this covered.
- Heali: Over 200 diseases? It’s all about you with this app’s custom plan. We tailor dietary approaches to help people deal with particular health challenges; think of it as a prescription for better health, but with food.
The human element in technological advancements
Recommendations alone often don’t solve long-term nutritional challenges. ML-driven recommendations face the same reality.
Good plans consider what people want and what’s best for the group. A plan only works if people actually use it.
Ethical considerations and data privacy in the age of ML-powered nutrition
Who is responsible for the very personal data we share about our bodies?
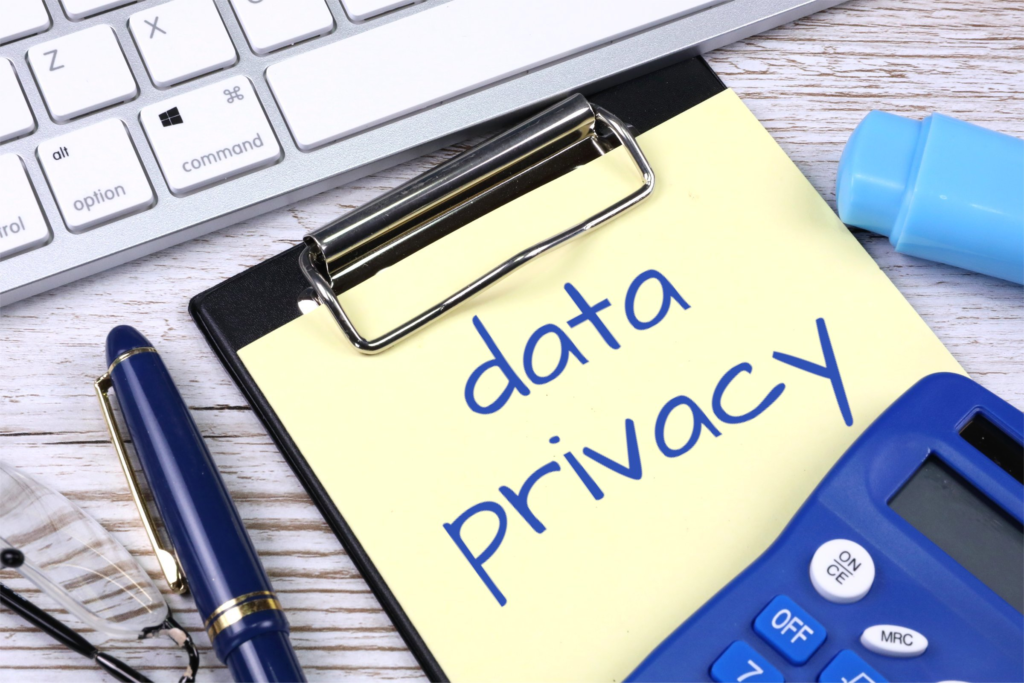
It’s about our data—the stuff that’s really personal and tied to our physical selves. Protecting this information is a top priority; it’s vital for security.
We must be cautious; rules like HIPAA govern how we handle user data. All data handling must adhere to these standards.
First impressions of the data matter a lot. Building good AI suggestions takes work.
Begin with accurate information; that’s the foundation of any successful data project. Think of it like building a house; you wouldn’t start without a solid base. To get the best analysis, include people from different walks of life. You’ll find their input to be priceless; it’s a game changer. Consider a medical diagnosis: multiple doctors provide more accurate diagnoses than one.
Beyond binary: Subgroup analysis and individualized treatment
Our bodies react differently to the world, even down to the foods we consume. Analyzing that data requires this perspective.
This may involve breaking down study participants into “subgroups.” Instead of treating nutritional data as a single continuum, subgroups might show different responses.
Blood tests give us a starting point for each person’s health data. The WHO created BMI readings to provide reference points for health status.
Subgroup analysis is all about sorting individuals. Think of it like separating different colored candies into their own piles. Think of it like sorting socks—but with people! Each person reacts differently to a nutritional process. It all comes down to machine learning in this step; it’s essential.
Individual risk predictions and ITRs.
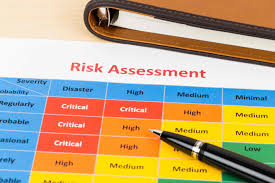
Assessments can also be made using separate predictions; this offers a fresh perspective. Measuring results? This tool? Works perfectly. Fair recommendations? Statistics are how we get there. Absolutely necessary. Think of it like this: we don’t want to unfairly favor certain things.
ITRs (Individual Treatment Rules) propose personalized solutions. These solutions will be different from person to person.
Picking the best option improves things. It’s that simple. Using computers to study ITR data has some real perks.
Reproducibility and replicability: This won’t be a walk in the park; there will be challenges.
Nutrition powered by machine learning will keep getting better. The scientists’ predictions? Better things are coming. They’re looking forward to advances in technology and computing capabilities; it’s only a matter of time.
Many studies so far focus on describing relationships between input data, like information from our smart watches, and nutritional goals. Reliable research means getting the same answer each time you test it using a similar process. Think of it like a recipe – follow the steps, get the same cake.
A diverse population will provide the necessary data; a significant amount of comparable data is needed for this study to be successful. Think of it like a puzzle needing many pieces. You’ve gotta test machine learning models extensively.
What constitutes a successful prediction rate for ML within these body types? General recommendations are shaped by this factor. Don’t forget: this is important. Don’t forget it.
Integrating social and cultural factors: A multidisciplinary approach
Machine learning applications in nutrition are still in early development. As techniques get better and are more thoroughly tested, outside influences will be considered.
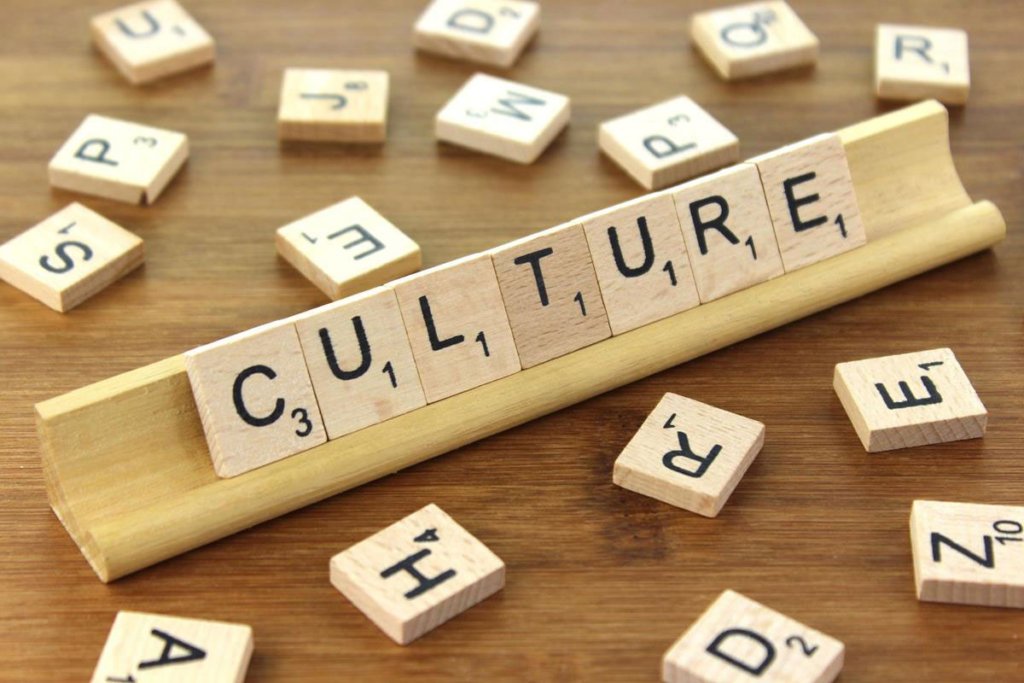
What we eat over the long term is shaped by our food customs and traditions. The food we eat and who we eat it with really matters. Social connections often happen around a table. Picture this: a big family dinner, a church service, a fun night out.
Culture, how much things cost, and what you like to eat all play a big part in making a diet plan that works long-term.
Personalizing your diet to fit your needs — how machine learning and you work together
Are you looking for answers, but feeling skeptical? Have traditional approaches failed to deliver the promised results?
It’s understandable, particularly if a previous plan didn’t bring the desired health improvements. Machine learning is all about personalization; it changes to fit you. This really works better.
Contribute by examining the details of your own information; gather it and then interpret the results. This is your part. We’ll base your nutritional plan on what you specifically need.
Imagine moving beyond one-size-fits-all dietary advice. Let’s use some smart computer programs and your favorite foods to figure this out.
Hence we sum up all the advice right here!
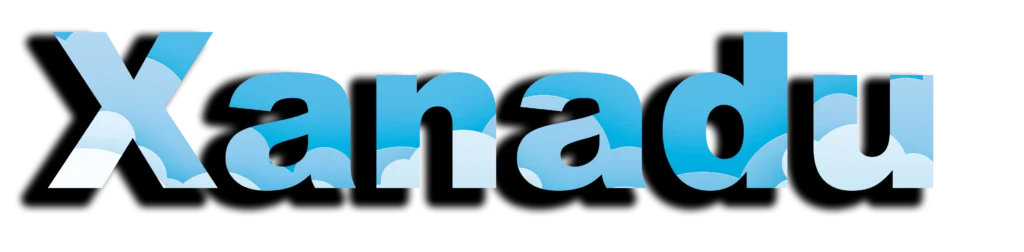
The best nutritional advice comes from a combination of scientific research, massive data sets, and technological breakthroughs; these are all incredibly important. Machine learning now brings this ability to each person.
The development process demands precision and planning; it’s a delicate matter. If a nutritional strategy, developed from careful use of only information about you, could help with your health concerns, would that make it a strategy you could more comfortably follow?
Think about all the new tools changing how we make and use food. Creating custom food is now possible thanks to machine learning; it allows for food solutions that are perfect for each person.
Be on the lookout here for the upcoming book “Reaching Xanadu.” And subscribe for more value in reaching your xanadu!
0 Comments